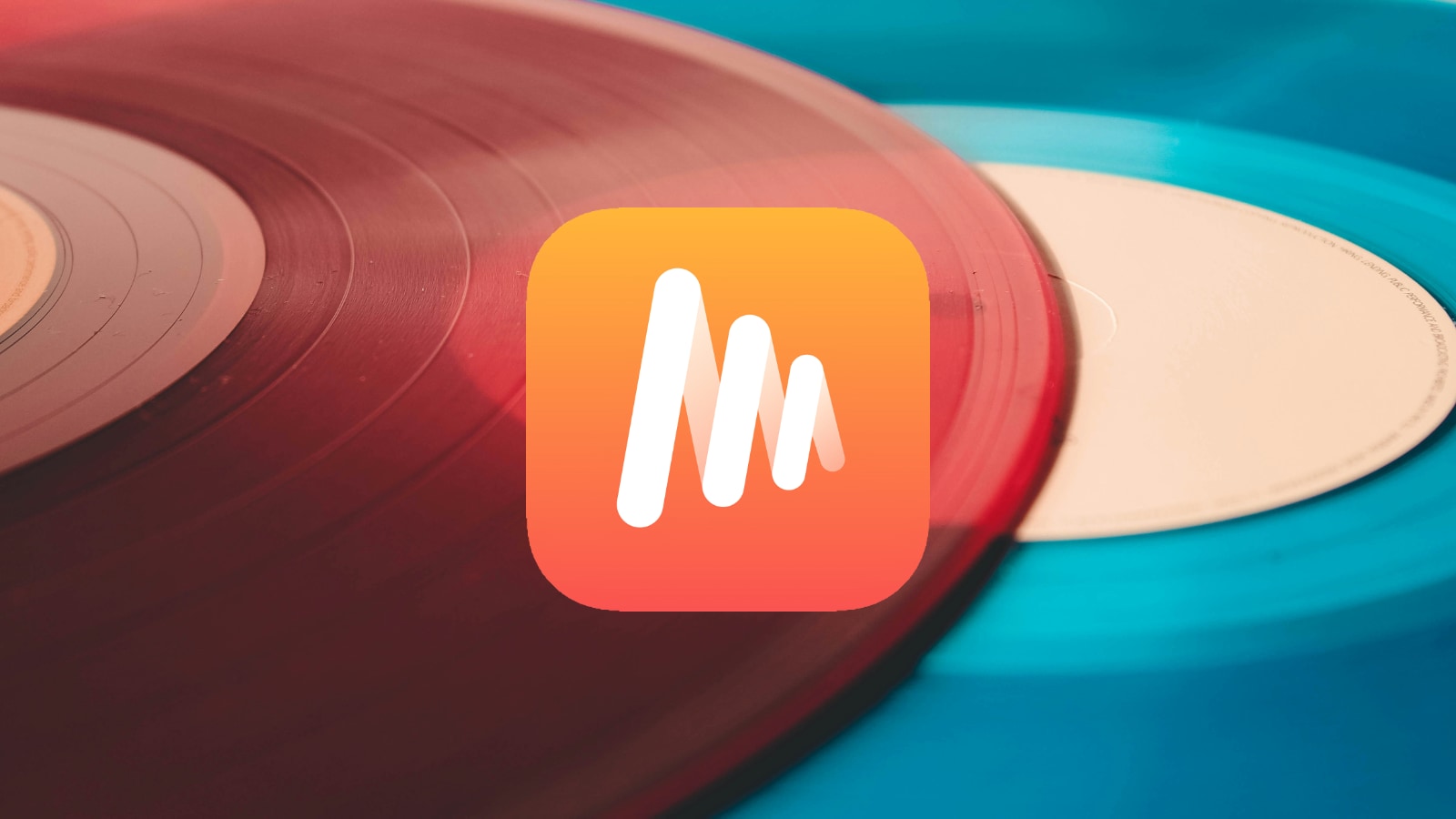
Artificial intelligence seems to be in the news more than ever before these days. From Deep Fakes to self-driving cars and facial recognition, there’s an AI application for just about every walk of life. For most people, the sudden presence of AI in daily life might seem rather sudden, but the metaphorical alignment of the stars has been going on for a long, long time. In order to understand where we might be going in an AI-centric future, it helps to understand a little of the AI evolution story.
Long before anyone built a real AI, human beings were imagining that such things would be possible. So the “soft” birth of AI, as with so many modern inventions, started on the pages of a storyteller.
Which was the first fictional depiction of artificial intelligence? I don’t think there can be a definitive answer because the definitions are a little fuzzy. In Greek Mythology we have the character of Galatea. A statue carved from ivory who then comes to life. Sounds like a prototypical story about a guy making a robot girlfriend, depending on how you look at it!
The Greeks had explicit automata in their stories as well, such as Talos. Which was basically a giant fighting robot from Pacific Rim, but classical. In fact, there are plenty of cultures with ancient tales that involve automata. Clearly, as a species, we’ve had a fascination with the idea of artificial, thinking machines for a long time.
More recently, in 1872, Samuel Butler (probably no relation) wrote the novel Erewhon, which was inspired by his short story Darwin Among the Machines. Butler is probably the first person to write about what we think of as “Strong” AI today. Since the machines in his stories develop consciousness.
If you’re a sci-fi fan, the story from here on becomes familiar to just about everyone. Rogue intelligent machines have been a plot device for decades in genre films and books. Films like The Terminator, Westworld, Wargames, and Tron have all cemented various ideas and myths about AI in pop media consciousness. For now, those superhuman strong AI are still just fantasy, but one day they may make the leap from imagination to reality.
In ancient times, there’s plenty of evidence that civilizations such as the Greeks had complex mechanical devices. The Antikythera mechanism is the only surviving physical example of this, but in their writings, the Greeks mentioned mechanical automata quite a lot. So it’s reasonable to think that they weren’t just something that existed in fiction.
Many cultures, such as the Jewish and Chinese folklore traditions, describe impressive automata.
The development of clockwork was a major advance. The Antikythera device is thought to have been an analogue computer, but clearly, centuries passed before the principles were rediscovered. In Europe, the 11th century saw clockwork in use for keeping time and even astronomical calculation.
Before the invention of vacuum tubes, the transistor and modern semiconductor technology to miniaturize logic devices to the nanoscale, clockwork was the most hi-tech thing around. One of the fathers of modern computing, Charles Babbage, proposed a device called the Analytical Engine. This was, on paper, a proper programmable computer. However, Babbage never managed to get all the funding and support in place to build the whole thing. Parts were made as a proof-of-concept, but it wouldn’t be until the Z3, finished in 1941, that the vision would be realized. The Z3 was an electromechanical computer and therefore not a Babbage Analytical engine. Yet it clearly drew on the same principles.
Humans have always looked to how nature solved problems for inspiration for our own inventions. The wing of a plane uses many of the principles you’ll find in the wing of a bird. So it makes sense that those who wanted to solve the issue of making artificial intelligence, you’d try to copy how real brains work.
The 20th century saw massive advances in our understanding of neurology. With the discovery of neural nets and how they use reinforcement for learning, it wasn’t long before AI pioneers tried to mimic them with artificial neural nets.
Marvin Minsky, who I mentioned earlier, revealed the SNARC to the world in 1952. That’s short for Stochastic Neural Analog Reinforcement Calculator. This is quite likely the world’s first-ever artificial neural net that could learn through reward. In other words, it was a machine that responded to operant conditioning, at least in principle.
This was an electromechanical device, not a digital computer, but AI researchers have been simulating (relatively) complex neural nets ever since. The neural net approach is just one branch of AI methods. However, today it’s seeing a massive revival in the form of Deep Learning. Which uses neural net simulation to do all sorts of things. DeepFakes, AI facial recognition and various machine vision projects all couldn’t exist without it.
Alan Turing is a pivotal figure in modern computing. A bona fide genius, Turing was instrumental in the very early days of digital computing, encryption, code-breaking, and artificial intelligence. It also a darn shame how he was treated by the government who he helped triumph in the Second World War.
His most famous contribution to the field of AI was the Turing Test. Turing was grappling with the problem of measuring milestones in AI development. After all, how can you tell if an AI is as smart as a human? We can’t even properly measure and compare the intelligence of actual humans. Turing contributes way, way more than just this test. Still, the Turing Test is something that is still thought-provoking today.
Something isn’t really an official field of study until someone spends money on holding a conference for it. So the Dartmouth Summer Research Project on Artificial Intelligence may very well mark the birth of AI as a formal field of research. It was quite a corker too, lasting eight weeks in 1956. Notably, Marvin Minsky attended the event. Minsky is arguably one of the biggest names in AI. Co-founding the MIT AI lab and advancing our understanding of cognitive science at the same time.
The Dartmouth project established the scope of AI research and worked from the premise that anything natural intelligence could do, you could program a computer to do as well. The computer might not exist yet and the programming method may be unresolved, but it could be done in principle. It must have been an amazing place to be at the time.
That massive research push-started at the Dartmouth conference kickstarted almost two decades of fervent AI research which lasted to about the mid-70s. You have to remember that the actual computers during this time were just giant pocket calculators, but the people working in AI did not have to be limited by the hardware of the time. Computer scientists, therefore, wrote programs that could solve all sorts of problems. Even if the actual hardware of the time had no hope of doing their work justice.
In fact, most of the AI technology revolutionizing the world today was developed in theory decades ago. It’s just that everyone is walking around with high-performance computers in their pockets now.
During this time lots and lots of money was being thrown at AI research. People were super-optimistic about how quickly AI and even Strong AI would become a reality. Heck, Marvin Minsky himself thought that human-level AI would arrive in the late 70s! However, it soon became clear that some fundamental problems were just too hard to solve. The same sorts of issues that face self-driving cars and machine vision systems today, that have only recently become effectively solved. As it became evident that the imminent arrival of an AI utopia wasn’t going to happen, the money started to dry up.
After making so much progress over the last 20 years, AI research ran into some pretty severe problems. There are two periods of time where pessimism about AI feasibility caused money for research to become scarce. In the field, they've been nicknamed the "AI Winters". The first happened from the mid- to late- 70s. With interest in AI picking up again during the 80s.
Then in the early 80s, there were major leaps in computing power and personal computers started to become a thing. Which again sparked optimism in the AI project. The second winter started in the mid-80s and ended just before the mid-90s. Many people reading this will, of course, remember that the 90s really kickstarted modern web-based computing. Computers went graphical, multimedia was the word of the decade and the computer performance road map was very exciting indeed.
These AI winters show us how short-sighted people are when facing tough problems, but they also demonstrate how hard you can run into seemingly impossible problems when it comes to AI.
While it's pretty simple to get a computer to solve sophisticated mathematics even the smartest mathematician would balk at, any healthy human can understand spoken language or recognize a face instantly. Plenty of people thought that these sorts of problems would be easy. However, it turns out that getting a machine to navigate 3D space, make sense of visual data or do anything even the simplest animals handle with ease is incredibly hard.
A lot of calculations around how much brute force computing power you'd need to crack these problems made them look literally impossible on paper. We've achieved many of these AI evolution milestones today because it turns out there are smarter methods that don't need as much raw power.
The day that Deep Blue beat chess Grandmaster Gary Kasparov is, in my opinion, the most pivotal moment in the history of AI Evolution. This was the first time that a Narrow AI was shown to be conclusively better than the best human being at one specific task.
By modern AI and computer hardware standards, Deep Blue us amazingly dumb and slow. The match against Kasparov where it finally made history and won was a pretty close call as well. Yet, none of that takes anything away from how important this moment was.
Deep Blue didn’t even use modern AI techniques such as Deep Learning. It was a brute force algorithm that overwhelmed Kasparov with pure speed and raw predictive capability. Still, it proved what many people thought would never be possible - a machine besting the very best humanity had to offer.
Deep Blue’s win over Kasparov was an amazing achievement, but Chess is a game that is relatively simple to quantify. Deep Blue basically used a powerful brute force approach to savagely explore the probability space of the game. Overwhelming its human opponent with sheer volume and speed. That can only get you so far and is a much smaller challenge than beating humans at the sorts of things we are actually good at.
The game of Jeopardy turns out to be a particularly hard nut to crack. If you’ve never watched this game show, here’s how it works.
Contestants must choose a category. Each category contains several cryptic clues phrased as the answer to a question. The contestant must answer with a question that would lead to the answer on-screen. It not only requires vast amounts of general knowledge, but it also requires symbolic thinking and complex language nuance.
Which is why IBM’s Watson computer is such a milestone in AI evolution. It competed with and beat humans at tasks that have long been thought of as uniquely human. Analyzing complex questions and coming up with creative answers is one of the last bastions of natural intelligence in humans. After Deep Blue beat Kasparov, the goal-posts moved. While no one can say that Watson understands anything its doing, the idea that machines will never perform at these complex language tasks has been smashed.
If you think Chess is a complex game, wait until you try Go. Thought to be the oldest ongoing board game in existence, this game offers a very different challenge to Chess. Chess has relatively few spaces and pieces. Although, there's plenty of complexity in the fact that the pieces are different.
In Go, even small boards have almost 400 points for pieces. So despite all the pieces being the same, as an AI problem experts thought it would be a long time before anyone wrote a program that could beat a human Go master. AlphaGo has evolved over several versions, with the latest named AlphaZero.
Apart from solving a fiendish problem in the form of Go mastery, the program is also special compared to Deep Blue thanks to it's more general-purpose nature. In other words, the program can apply its intelligence to problems other than Go. So no matter which way you look at it, AlphaGo is both an AI milestone comes earlier than expected and possibly a future ancestor of the first Strong AI.
As we move further into this century, it's going to become almost impossible to live a life that AI won't touch in one way or another. AI is being used to solve a new complex problem every day. Of course, AI abuse might lead to entirely new problems we didn't have before, but the advantages are too many to avoid the inevitable march of progress. Now the exciting part is getting to experience whatever the next step in AI evolution will be.
What is the most amazing part of AI Evolution to you? Let us know down below in the comments. Lastly, we’d like to ask you to share this article online. And don’t forget that you can follow TechNadu on Facebook and Twitter. Thanks!